The risks linked to the mass use of artificial intelligence lie chiefly in the reproduction of human biases and stereotypes. To create ethical AI, developers must create data-driven, trustworthy AI solutions by design in a technical, legal and humanistic way.
Science fiction is replete with tales of rebellious AIs, where machines act according to their own nefarious value systems. Remember HAL 9000 in Space Odyssey or Skynet in Terminator? But in real life, AI systems and algorithms have been widely integrated into everyday activities such as shopping online, applying for loans or consulting social networks. Although cases of renegade AI happily remain the fantasy of authors, AI drifts and malfunctions have become commonplace, and they often have very real consequences.
Fighting against stereotypes with ethics
We’ve all seen the images generated by AIs like Dall-E 2. It’s innocent fun to create a picture of a teddy bear listening to music underwater. But are you aware that the algorithms used perpetuate gender stereotypes by over-representing men in images of doctors, pilots and CEOs, while over-representing women in images of nurses, flight attendants and secretaries?
Given the massive number of everyday decisions delegated to AI, we cannot ignore the ethical questions raised by its use. AI remains a market and a technology that is still poorly legislated (although the European Commission is actively working on future regulations). It is therefore the responsibility of every individual to protect users.
The challenge for AI is to create trustworthy systems by design that meet the ethical expectations of our society. It is imperative that we prepare, beginning now, to design and maintain trusted AI systems. This is a vast undertaking: ethics is a subject that is built throughout the entire lifecycle of a product.
Artificial intelligence that reproduces human errors
Artificial Intelligence is a set of theories and techniques that allow a machine to make decisions when faced with a predefined situation. In other words, it simulates human intelligence. Such programs can either be deterministic, where successive rules are applied according to a decision-making scheme, or probabilistic, where, after learning from similar past situations, the program can infer what the ‘right’ decision should look like. This is also called machine learning.
Regardless of the program type, AI is the automatization of decision-making. The ethical risks do not reside in the fact that AI may automate badly, but on the contrary, that it perfectly mimics human decisions, with its burden of errors and biases. Many of these dysfunctions come from the cultural context in which the AI was developed, when it replicates the historical value systems of a society (gender or ethnic inequality, for example) or those of its designers (education, political sensitivity, religious practices, etc.).
These biases are particularly prevalent in machine learning algorithms since they are trained on legacy databases, inferring the future from the past.
The essential questions linked to conceiving and developing a trustworthy AI lifecycle concern both technical and ethical issues, such as how to reliably detect possible biases in an AI? How to interpret a model’s results? Can we measure the evolution of an AI’s performance? What safety guarantees can we provide? Can we react to a drift? This non-exhaustive list reflects the diversity of the challenges to be met during the production of an AI.
Using AI to correct AI biases
As AI models have evolved from concepts to products, many technical solutions have been created to facilitate the automation and deployment of AI systems. Recently, the number of available technical solutions has exploded, creating a rich ecosystem fueled by constant innovation from start-ups and industry leaders alike. Notable examples are the AI Infrastructure Alliance and the MLOps Community, both of which Artefact is a member. Finding the right tool for the right task is therefore becoming more important, and more difficult.
At the same time, an equally vibrant ecosystem of consulting firms has developed to support their clients in making these strategic choices.
Many of these technical solutions exist in open source and are freely available to all. These toolkits can detect and measure biases all along the data processing chain: from collection to exploitation, including transformation and modeling. These solutions must be implemented throughout the product lifecycle, both at the design and development stage as well as in production, in order to ensure permanent correction. There are three particular areas that can be addressed with technical solutions.
“Biases don’t come from data scientists, they come from datasets.”
Correcting past biases in AI
Biases do not originate with data scientists, but with datasets. This is why it’s necessary to explore and think deeply about datasets first. Biases can be both technical (omitted variables, database or selection problems) and social (economic, cognitive, emotional).
To rectify training sets, it might seem logical to erase all traces of sensitive data. However, this would be as useless as it would be dangerous: on the one hand, because non-sensitive data can be insidiously correlated with sensitive data, and on the other because this type of shortcut encourages limiting regular checks on data and processes.
To detect these biases, one must be aware that they exist and know what to look for. It is important to understand the context of the data in order to interpret it correctly. A single program cannot identify bias, hence the importance of diverse teams of data scientists.
It is by thinking critically about the different metrics associated with an AI model that we can identify and rectify problems. Consider a model that needs to detect a disease present in 1% of the population. If the model always predicts that the person is healthy, the model will have an accuracy rate of 99%. Without context, this is an excellent score. Yet this model is useless.
Using basic, univariate and bivariate dataset statistics can identify some biases. For example, is each age group represented equally enough? One solution might be to change continuous data into categorical data. Correlation matrices can also validate links between two related variables. All this upstream work is crucial to ensure that the models are trained on quality datasets. In a nutshell: “Garbage in, garbage out”.
Explaining the results of a model
High-performing AI systems, such as artificial neural networks, are very efficient and used in multiple use cases. However, they are difficult to interpret, which is why they are often referred to as “blackbox models.” The great challenge of machine learning is to identify and explain the cause of bias rather than to dismiss its consequences. There is a trade-off between explainability and accuracy. Some algorithms like decision trees are very explainable, but less useful for more complex predictions.
The field of Explainable AI (Ex-AI) attempts to solve this problem by developing methods and algorithms that increase the explainability of systems. One can either explore global understanding, which explains how an AI works on the global population, or local understanding, which explains how the algorithm works on a specific example. The latter is mandated by the GDPR because to be transparent, an AI must be able to explain why the model made a certain decision for a particular person/object/data line. It is important to look at both global and local understanding to understand how the model behaves.
To do this, data scientists can look at the significance variables of the model. For example, it isn’t normal for a recruitment model to have gender as an important variable. They can also check metrics such as accuracy (number of well predicted positives = true positive divided by all predicted positives = true positive + false positive), F1 score (summarizes the values of accuracy and recall in a single metric), or recall (number of well predicted positives = true positive divided by all positives = true positive + false negative), in relation to a variable (e.g. accuracy for women vs. men).
“The great challenge of machine learning is to identify and explain the cause of bias rather than to dismiss its consequences.”
Improving the robustness of a model
Sources of malfunction in Artificial Intelligence models can be accidental, when data is corrupted, or intentional, when caused by hackers for example. Both of these sources of bias lead AI models into error by providing incorrect predictions or results. Some solutions enable the evaluation, defense, and verification of machine learning models and applications against conflicting threats that might target the data (data poisoning), the model (model leakage) or the underlying infrastructure, both hardware and software.
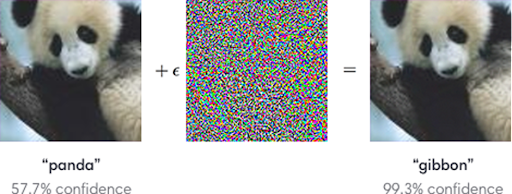
Photo source: https://arxiv.org/pdf/1412.6572.pdf
Simply by superimposing a ‘noise’ image on a normal image, a classifier can be led to misclassify a panda as a gibbon. The difference is imperceptible to the human eye, but this technique is well documented as being able to mislead AI models.
Developing less human, but more humanistic systems
The notion of trustworthy AI cannot be reduced to its legal and technical conception. Human and organizational aspects are crucial to the success of an ethical approach to AI. Ethical charters and improvement solutions must be known by all stakeholders, applied throughout the creation process, and followed over time. This requires a transformation of the organization’s culture so as to deeply integrate ethical issues and approaches, guarantee the sustainability of solutions and contribute to ethical AI by design.
AI in itself is neither ethical nor unethical. It is only the way the system is trained or how it is used that is ethical or not. The true ethical risk linked to the massive use of AI is therefore not that algorithms might revolt. On the contrary, the risk arises precisely when AI behaves exactly as we have asked it to do, mimicking our biases, repeating our mistakes, and reinforcing our uncertainties and imprecisions.
“The risk arises precisely when AI behaves exactly as we have asked it to do.”
Being proactive on ethical AI
This subject is close to our hearts at Artefact, which is why we’ve dedicated teams to it, and have developed specific support on governance development, technical solution implementation, and AI strategy consulting.
We work in close collaboration with the academic world, as a partner of the Good in Tech chair, co-founded by the Institut Mines-Télécom and Sciences Po, to reduce the gap between research and practice. Artefact has also received the Responsible and Trusted AI label, awarded by independent association Labelia Labs, which guarantees a high level of maturity on the subject of responsible and trustworthy AI.
The issue of ethics concerns us all, and it is essential to be proactive on the subject; the adoption of ethical behavior should not merely be a reaction. Artificial intelligence is going through an evolutive period, where its processes and ethos are in the process of being defined. In a field that is not yet regulated, it is up to those who are playing an active role to take the lead. As developers, consultants, and managers, it is our responsibility to meet the expectations of everyone involved – not only customers and service users, but society in general – and to use the power of AI to create a better world.