Data Science & MLOps
We apply MLOps to industrialize reliable products at speed.
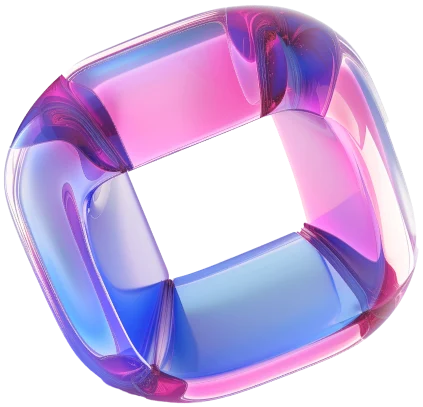
Our MLOps methodology delivers scalable AI models quickly and effectively.
Machine Learning (ML) is a form of AI that lets a system continuously learn from data through virtuous algorithms rather than explicit programming. It offers potential value for companies that use data to better understand the subtle changes in their customers’ behaviours, preferences and levels of satisfaction.
But despite these capabilities, machine learning also comes with challenges and risks. Firstly, complex ML models need to be regularly refreshed, which can incur high production deployment costs. Secondly, if data quality is not closely monitored, the AI can quickly suffer from performance drift and bias. To solve these challenges, we close the gap between Proofs of Concepts (POC) and Production by applying our Machine Learning Operations (MLOps) methodology to all of our Data and AI projects.
Our methodology is inspired by the DevOps approach used by the most innovative software companies, combining software development (Dev) and IT operations (Ops).
It aims to shorten the systems development life cycle and provide continuous delivery with high software quality.
Our MLOps approach helps companies seamlessly industrialize and scale their AI products.
The traditional approach of using Machine Learning’s capabilities has several drawbacks:
Data Scientists hardly foresee production constraints. They work in silos without interaction with software or data engineers. Their one-shot analyses in Python notebooks need to be reworked by downstream engineers to fit industrialization requirements. This induces slowness and reduces time to market.
A lack of agility, which leads to high operational risk. In case the produced algorithms reveal themselves biased, unstable or prone to customer dissatisfaction, companies will not be able to respond in an acceptable time frame.
We think “product first” to help companies progress their AI assets smoothly to production while anticipating industrialization constraints and risks. Our MLOps model is based on a solid ecosystem, and we apply the same processes for every AI project we deliver, from POC to product deployment.
A succesful MLOps approach to accelerate the data & AI transformation of organizations
A solid monitoring stack.
We test all data, features and models before every new release to prevent quality or performance drift.
Our data, models and learning experiments are all versioned and logged to ensure fast rollback in case of production incidents.
A resilient machine learning infrastructure.
We embed all Machine Learning assets (code, data, models) in a Continuous Integration and Continuous Delivery pipeline (CICD) to secure fast and seamless rollouts to production.
A strong collaboration culture.
We ensure all stakeholders work on the same canvas and apply software engineering best practices to Data Science projects (versioning, deployment environments, testing).
Read our Data Science blog post explaining how we apply MLOPS for our clients.
Our Data Scientists are passionate about developing industrialized solutions and tackling complex challenges
Data Science is a challenging field, with ever evolving methodologies and technological advancements. Our team stays on top of these changes, always keeping an eye on adapting to new business needs.
With our machine learning expertises, highly skilled and motivated data experts combined with a unique collaboration methodology and a ‘product first’ mindset, our Data Science team will help you solve your most challenging problems.
We are pragmatic and results focused engineers: we infuse our work with state-of-the-art algorithms keeping as priority the ease of implementation and short term returns on investment.
We solve problems.
How can you improve your customer lifetime value? Better understand a customer journey? How do you predict the movement of a brand new product or find new consumer trends into several millions of social networks posts?
Our data scientists have a proven track record of problem solving issues for several large companies, across diverse sectors.
We work with retail, luxury, financial services, pharmaceuticals, private equity or even telecommunication companies, to leverage machine learning and analytics to create impactful solutions for our clients.
At Artefact, we have direct interactions with the end-user of the solutions we implement. This allows us to do data science not just for the beauty of it, but to answer real needs. Getting immediate feedback on the added value it can bring, the challenge that needs to be answered, and how your product is being used allows you to really focus on what matters and to develop a solution that will be of help to the user.”
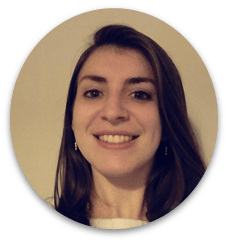
Louise, Data Scientist
We work in feature teams to break silos.
In most organizations, data science teams work in silos. Their services do not scale across the full value chain and, all too often, they create ‘black box’ solutions that very few people can understand and maintain.
At Artefact, we break these silos to reach common business goals. Our data scientists work collaboratively, in feature teams, alongside stakeholders such as business owners, software engineers, DevOps, and UX designers to ensure all objectives and priorities are taken into account.
Working with Product Owners, Software Engineers and fellow Data Scientists is a truly enriching experience. Responsibilities within the team are much clearer, meaning that the Data Scientists can free a lot more time to focus on technical tasks, while staying updated about all aspects of the project. Respecting agile methodology best practices also provides more structure, ensuring that we always prioritize what will yield the most value.
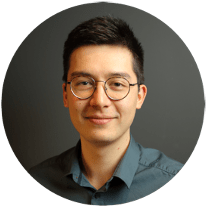
Paul, Data Scientist
We think “Product” first!
We do not stop at the POC (proof of concept) stage, we always go further until industrialisation and deliver impactful and resilient products.
Our Data Scientists are committed to delivering industrialised softwares, deploying a valuable and reliable solution is our first priority, well before fine-tuning our AI algorithms. Building sound foundations in our project allows us to deploy seamlessly and quickly new features for an increased value.
There are usually many possible innovative solutions to a problem, finding the one that is optimal in the context of our clients’ needs, constraints and technical stacks is where the subtlety lies. We usually go further than the proof of concept in an isolated environment. Putting a model into production is a complex task that demands best practices in MLOps, rigorous monitoring and evaluation in order to ensure and maintain the best level of performance while addressing the technical and ethical implications.
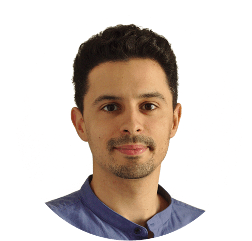
Karim, Data Scientist
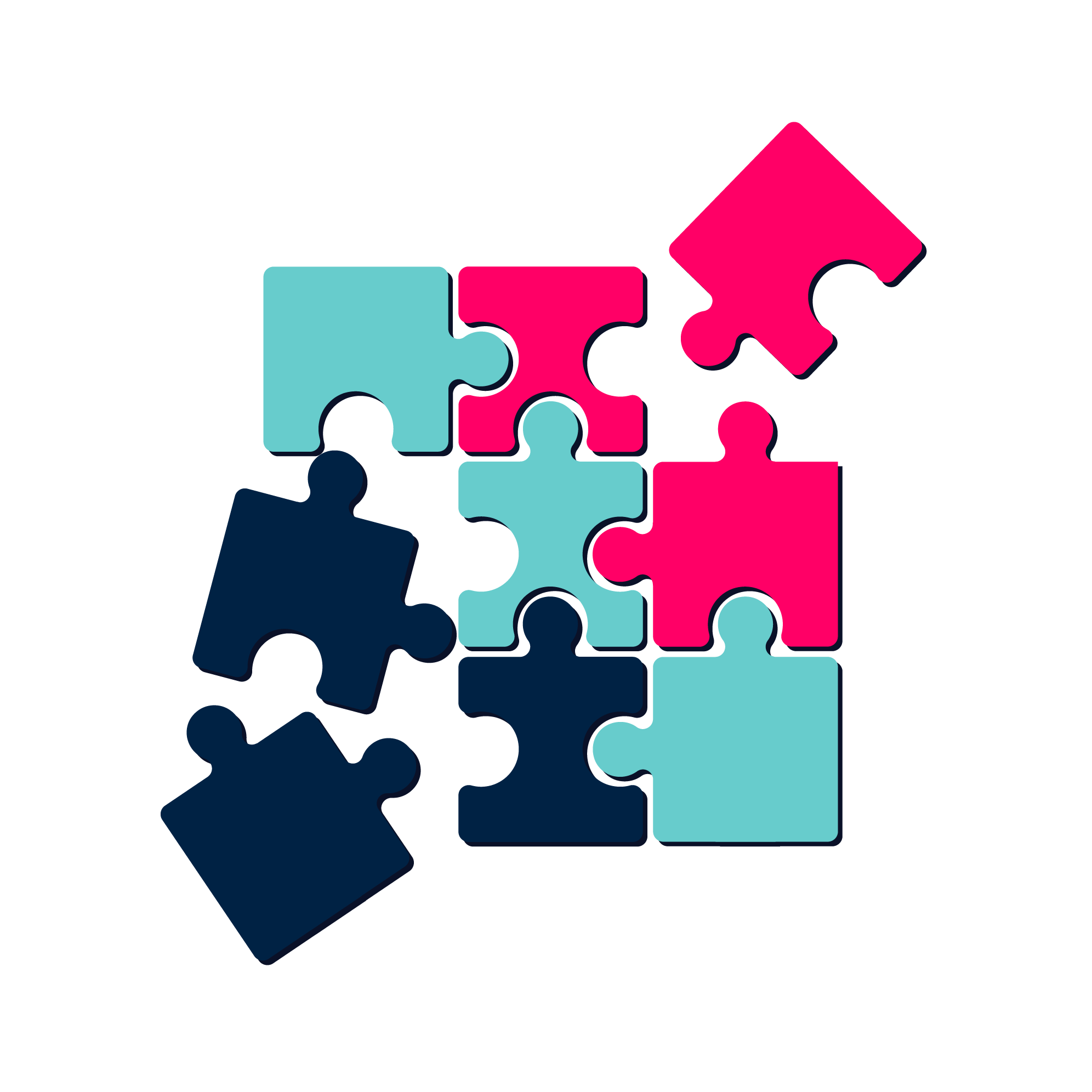
Our strength,
Our expertises.
Data science sits at the intersection of applied domain knowledge, mathematics, statistics and computer science.
To nurture our R&D effort, better answer our client needs and apply the latest AI advancements in our projects, we have created, at Artefact, a set of task forces specialized into each machine learning subfield.
What is the life of a Data Scientist at Artefact ?
Working on complex and challenging missions
From supply chain to customer services, our data scientists have been working on various challenging subjects: predicting volume of calls in call centers, automating responses to customers’ requests, detecting beauty or luxury consumption trends or even helping physicians detect cancerous cells in X-rays.
Working at Artefact is also an opportunity for tech savvy engineers to develop their business understanding and better grasp the subtleties of most major industries. All our data scientists are in charge of crafting tailor made solutions that will answer very specialized business challenges and are working hand in hand with our c-level clients to foster adoption and embed business logic into smart AI products.
One of the most exciting things about working at Artefact is the variety of subjects we tackle. The term data science can cover a wide range of skills in the domains of natural language processing, forecasting or optimization to mention only a few, so each new mission brings its share of new algorithms to test and new technologies to experiment with. But picking the right model for the job is not the only responsibility of a data scientist: we need a very good understanding of the business stakes to know where we can bring the most value, which means we work closely with consultants and with our client. In order for our work to be useful in the long term, we also coordinate with software engineers and apply software best practices to turn our insights into a product.
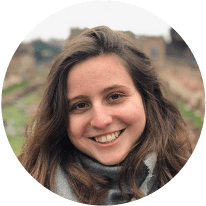
Ombeline, Data Scientist
A dedicated training track to help our teams grow
Data science is a constantly changing field and we have our heart set on continuously training our engineers.
Data Scientists at Artefact can benefit from a large set of internal and external training, chosen carefully by our training department, helping them reach the technological frontier.
We provide:
– Machine learning trainings (NLP, Forecasting, Computer Vision, ML Operations…)
– Access to Cloud certifications (GCP, Azure, AWS)
– Soft skills trainings (Oral and written presentation, negotiation, project management)
Training does not stop there: a typical data scientist’s week is infused with opportunities to learn. As we like to say “Feedback is a gift” and our culture is built around tech events such as our TechTex where we share our latest projects successes and failures or our Code Base Committee (CBC) where our projects code is challenged by our guru coders!
A tech company within a consulting company
Artefact is a consulting company, but the DS team is first and foremost a tech department:
– We leverage the latest model and ML libraries such as Sklearn, FastAI, CatBoost, Prophet, Spacy, BERT and its variants (CamemBERT, DistilBERT, …) and many more
– We are multi cloud and certified premium customer in the biggest clouds such as GCP, Azure or AWS
– We build AI products leveraging ML Ops frameworks and utilities such as Docker, Kubernetes, Kedro, ML Flow, Great Expectations, and so many more!
We promote R&D within our teams in order to stay up to date with the latest releases in the tech world.
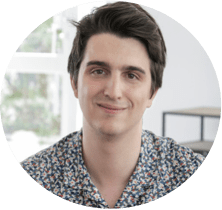
Robin Doumerc, Global CTO Artefact
Our field has been in constant evolution in recent years, with new algorithms, methods and implementations. Keeping up to date in this ever-changing ecosystem can be a daunting task if you’re alone. Therefore, continuous training as a team is an essential part of our life at Artefact, either through internal projects where they can try the latest tech in a problem they encounter everyday, or dedicated time during our monthly training days. Allowing our Data Scientists to continue learning on cutting-edge subjects ensures that we maintain their curiosity, but also partly their well-being within the team.
Join Us
Our team has a proven track record of missions involving propensity modeling and recommendation systems. Contact us and quickly get in touch with one of our experts should you wish to know more about our expertises.
If you’d like to join us, please follow our careers webpage .
Medium blog articles by our tech experts
Assortment Optimization with discrete choice models in Python
Assortment optimization is a critical process in retail that involves curating the ideal mix of products to meet consumer demand while taking into account the many logistics...
Is Preference Alignment Always the Best Option to Enhance LLM-Based Translation? An Empirical Analysis
Neural metrics for machine translation (MT) evaluation have become increasingly prominent due to their superior correlation with human judgments compared to traditional lexical metrics
Choice-Learn: Large-scale choice modeling for operational contexts through the lens of machine learning
Discrete choice models aim at predicting choice decisions made by individuals from a menu of alternatives, called an assortment. Well-known use cases include predicting a...
The era of generative AI: What’s changing
The abundance and diversity of responses to ChatGPT and other generative AIs, whether skeptical or enthusiastic, demonstrate the changes they're bringing about and the impact...
How Artefact managed to develop a fair yet simple career system for software engineers
In today’s dynamic and ever-evolving tech industry, a career track can often feel like a winding path through a dense forest of opportunities. With rapid...
Why you need LLMOps
This article introduces LLMOps, a specialised branch merging DevOps and MLOps for managing the challenges posed by Large Language Models (LLMs)...
Unleashing the Power of LangChain Expression Language (LCEL): from proof of concept to production
LangChain has become one of the most used Python library to interact with LLMs in less than a year, but LangChain was mostly a library...
How we handled profile ID reconciliation using Treasure Data Unification and SQL
In this article we explain the challenges of ID reconciliation and demonstrate our approach to create a unified profile ID in Customer Data Platform, specifically...
Snowflake’s Snowday ’23: Snowballing into Data Science Success
As we reflect on the insights shared during the ‘Snowday’ event on November 1st and 2nd, a cascade of exciting revelations about the future of...
How we interview and hire software engineers at Artefact
We go through the skills we are looking for, the different steps of the process, and the commitments we make to all candidates.
Encoding categorical features in forecasting: are we all doing it wrong?
We propose a novel method for encoding categorical features specifically tailored for forecasting applications.
How we deployed a simple wildlife monitoring system on Google Cloud
We collaborated with Smart Parks, a Dutch company that provides advanced sensor solutions to conserve endangered wildlife...