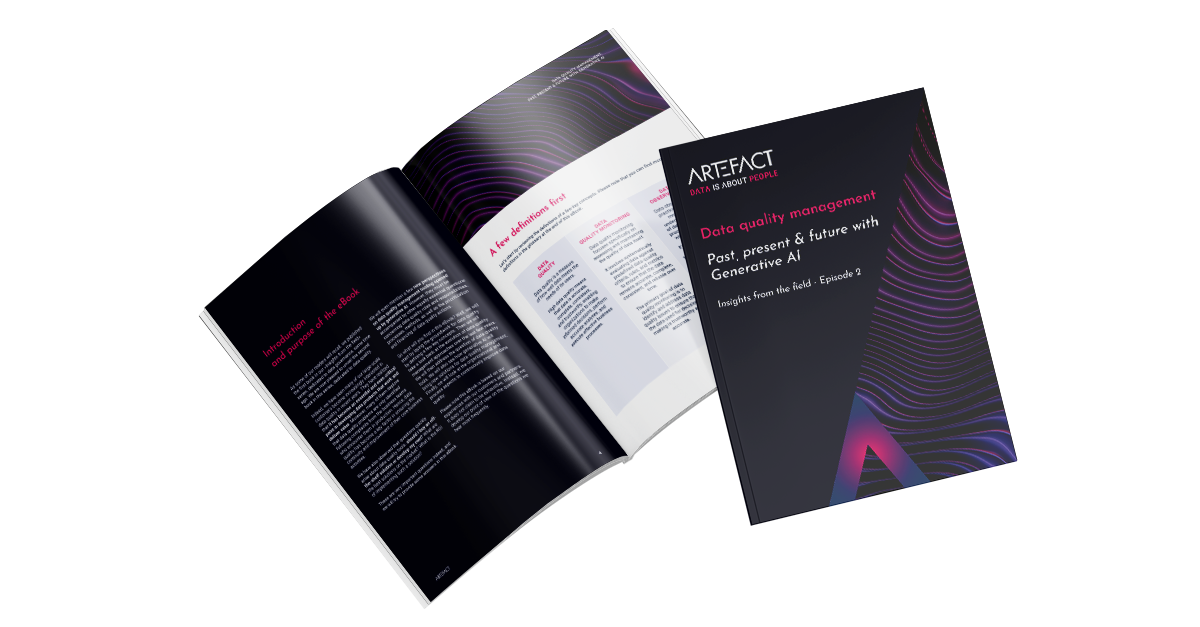
As some of our readers will recall, we published the first book in our «Insights from the field» series, dedicated to data governance, some time ago. We are now pleased to unveil the second book in this series, dedicated to data quality. Indeed, we have seen many of our large-scale
customers become increasingly interested in data quality in recent years. They have realized that it has become an essential and even critical
point in developing data products that work and deliver value. Moreover, many of them deplore that data quality problems are only identified
following complaints from the business teams who encounter them in production. Hence, data quality has become a key factor in ensuring the continuity and improvement of their core business activities. We have also observed that questions quickly arise about data quality tools: should I buy an off-the-shelf solution or develop my own? What are the best solutions on the market? What is the ROI of implementing such a solution?
We will even mention a few new perspectives on data quality management tooling opened up by generative AI. However, they must be accompanied by other equally essential questions concerning data quality roles and responsibilities, processes, methods, as well as the prioritization and financing of data quality actions. So what will you find in this eBook? Well, we will start by laying the groundwork for data quality by defining a few key concepts. Then we will take a look back at the evolution of data quality management approaches over the last few years. We will then address the question of data quality tools. We will also see how generative AI will bring new solutions for data quality management. Finally, we will look at the organizational and process aspects to continuously improve data quality.
Please note this eBook is based on our experiences with our customers and partners. It does not claim to be exhaustive. Instead, we develop our point of view on the questions we hear most frequently.
Table of contents
- Introduction and purpose of the eBook
- A few definitions first
- What are the common business impacts of poor data quality?
- How has the approach of data quality management evolved in recent years?
- Which tools for data quality management?
- How will Generative AI enhance data quality management?
- What operating models should be implemented for data quality management?
- In summary: Three key message to remember
- Glossary
Share this report