Data Science & MLOps
We apply MLOps to industrialize reliable products at speed.
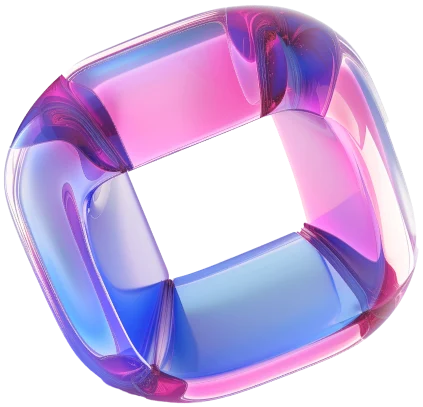
Our MLOps methodology delivers scalable AI models quickly and effectively.
Machine Learning (ML) is a form of AI that lets a system continuously learn from data through virtuous algorithms rather than explicit programming. It offers potential value for companies that use data to better understand the subtle changes in their customers’ behaviours, preferences and levels of satisfaction.
But despite these capabilities, machine learning also comes with challenges and risks. Firstly, complex ML models need to be regularly refreshed, which can incur high production deployment costs. Secondly, if data quality is not closely monitored, the AI can quickly suffer from performance drift and bias. To solve these challenges, we close the gap between Proofs of Concepts (POC) and Production by applying our Machine Learning Operations (MLOps) methodology to all of our Data and AI projects.
Our methodology is inspired by the DevOps approach used by the most innovative software companies, combining software development (Dev) and IT operations (Ops).
It aims to shorten the systems development life cycle and provide continuous delivery with high software quality.
Our MLOps approach helps companies seamlessly industrialize and scale their AI products.
使用机器学习的能力的传统方法有几个缺点。
Data Scientists hardly foresee production constraints. They work in silos without interaction with software or data engineers. Their one-shot analyses in Python notebooks need to be reworked by downstream engineers to fit industrialization requirements. This induces slowness and reduces time to market.
缺乏敏捷性,导致高运营风险。如果产生的算法显示自己有偏见、不稳定或容易引起客户的不满,公司将无法在可接受的时间范围内作出反应。
We think “product first” to help companies progress their AI assets smoothly to production while anticipating industrialization constraints and risks. Our MLOps model is based on a solid ecosystem, and we apply the same processes for every AI project we deliver, from POC to product deployment.
A succesful MLOps approach to accelerate the data & AI transformation of organizations
坚实的监测堆栈
我们在每次发布新版本之前都会测试所有的数据、功能和模型,以防止质量或性能漂移。
我们的数据、模型和学习实验都是有版本的,并记录在案,以确保在发生生产事故时快速回滚。
弹性的机器学习基础设施
我们将所有机器学习资产(代码、数据、模型)嵌入持续集成和持续交付管道(CICD)中,以确保快速和无缝地推出到生产中。
强大的合作文化
我们确保所有利益相关者在同一画布上工作,并将软件工程最佳实践应用于数据科学项目(版本、部署环境、测试)。
阅读我们的数据科学博客文章,解释我们如何为客户应用MLOPS。
Our Data Scientists are passionate about developing industrialized solutions and tackling complex challenges
数据科学是一个具有挑战性的领域,有着不断发展的方法论和技术进步。我们的团队紧跟这些变化,始终关注着适应新的业务需求。
凭借我们的机器学习专业知识、高技能和积极的数据专家,结合独特的合作方法和 "产品第一 "的思维方式,我们的数据科学团队将帮助您解决最具挑战性的问题。
我们是务实和注重结果的工程师:我们在工作中注入了最先进的算法,并把易于实施和短期的投资回报作为优先事项。
我们解决问题
如何提高你的客户终身价值?更好地理解一个客户的旅程?你如何预测一个全新产品的动向,或者在几百万个社交网络帖子中找到新的消费趋势?
Our data scientists have a proven track record of problem solving issues for several large companies, across diverse sectors.
We work with retail, luxury, financial services, pharmaceuticals, private equity or even telecommunication companies, to leverage machine learning and analytics to create impactful solutions for our clients.
在Artefact ,我们与我们所实施的解决方案的终端用户有直接的互动。这使我们在做数据科学时不仅仅是为了美,而是为了满足真正的需求。获得关于它能带来的附加值、需要回答的挑战以及你的产品如何被使用的即时反馈,使你能够真正专注于重要的事情,并开发出对用户有帮助的解决方案。"
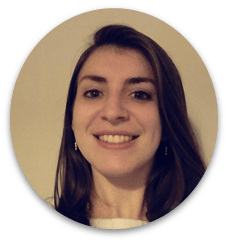
Louise,数据科学家
我们在全能团队中工作,以打破行业壁垒。
In most organizations, data science teams work in silos. Their services do not scale across the full value chain and, all too often, they create ‘black box’ solutions that very few people can understand and maintain.
在Artefact ,我们打破这些壁垒,以达到共同的业务目标。我们的数据科学家在功能团队中与利益相关者(如业务所有者、软件工程师、开发流程和用户体验设计师)合作,以确保所有目标和优先事项都得到考虑。
与产品拥有者、软件工程师和其他数据科学家一起工作是一种真正丰富的经验。团队中的职责更加明确,这意味着数据科学家可以腾出更多的时间来专注于技术任务,同时保持对项目各方面的更新。尊重敏捷方法论的最佳实践也提供了更多的结构,确保我们总是优先考虑能产生最大价值的东西。
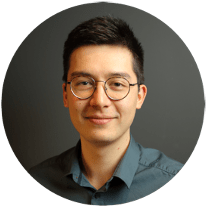
Paul,数据科学家
We think “Product” first!
我们不会止步于POC(概念验证)阶段,我们总是更进一步,直到产业化,并提供有影响力和弹性的产品。
我们的数据科学家致力于提供工业化的软件,部署一个有价值和可靠的解决方案是我们的首要任务,远远早于微调我们的人工智能算法。在我们的项目中建立健全的基础,使我们能够无缝和快速地部署新的功能,以提高价值。
一个问题通常有许多可能的创新解决方案,在我们客户的需求、限制和技术堆栈的背景下,找到一个最佳的解决方案是微妙的地方。我们通常比在一个孤立的环境中进行概念验证更进一步。将一个模型投入生产是一项复杂的任务,需要MLOps的最佳实践,严格的监测和评估,以确保并保持最佳的性能水平,同时解决技术和道德方面的问题。
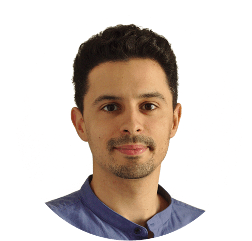
Karim,数据科学家
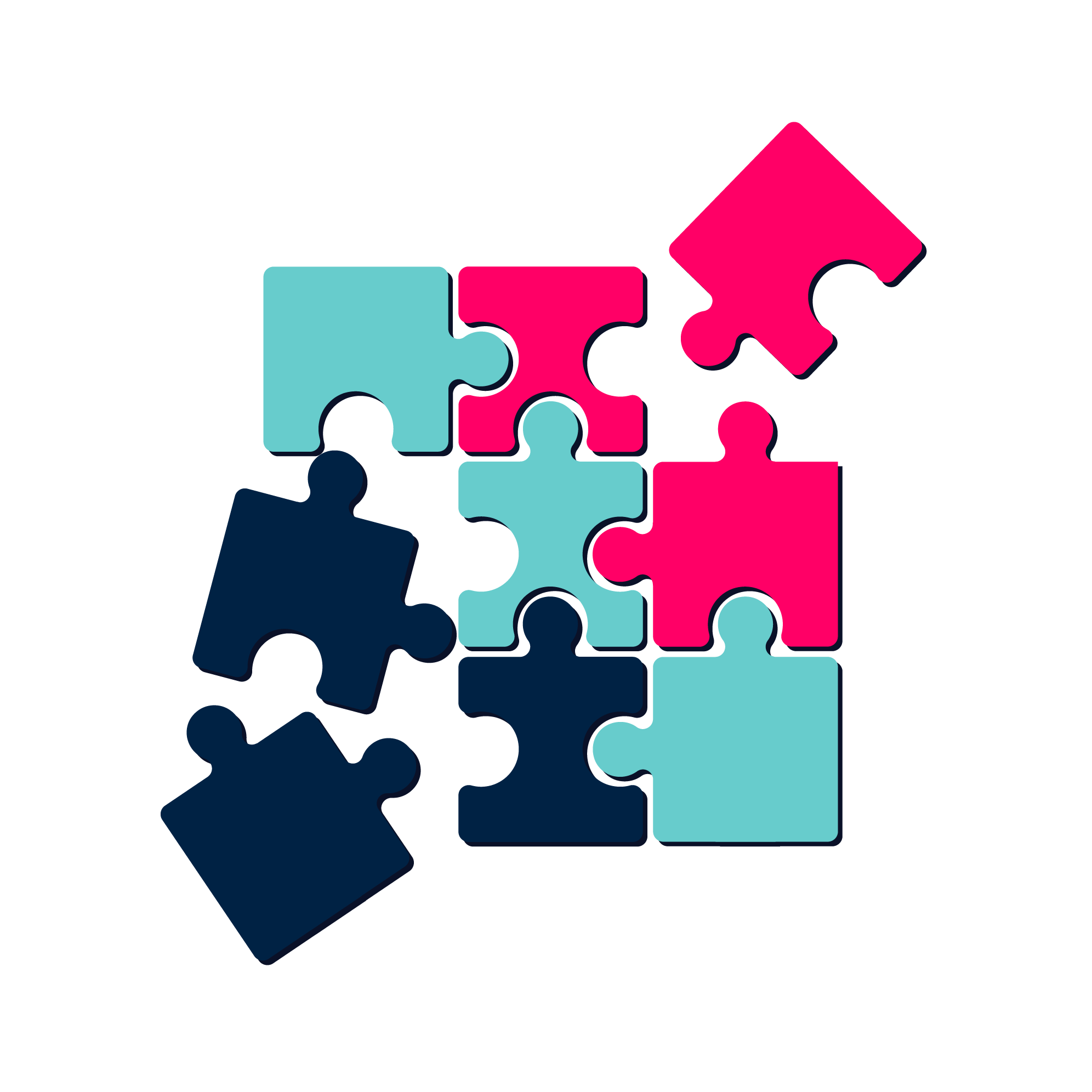
Our strength,
Our expertises.
数据科学位于应用领域知识、数学、统计学和计算机科学的交叉点。
为了培养我们的研发工作,更好地回答客户的需求,并在我们的项目中应用最新的人工智能进展,我们在Artefact ,建立了一套专门针对每个机器学习子领域的任务组。
Artefact 数据科学家的生活是怎样的?
从事复杂和具有挑战性的任务
从供应链到客户服务,我们的数据科学家一直在研究各种具有挑战性的课题:预测呼叫中心的通话量,自动回应客户的要求,检测美容或奢侈品消费趋势,甚至帮助医生检测X射线中的癌细胞。
在Artefact ,对于精通技术的工程师来说,也是一个发展他们的业务理解和更好地掌握大多数主要行业的微妙之处的机会。我们所有的数据科学家都负责精心设计定制的解决方案,以回答非常专业的业务挑战,并与我们的C级客户携手合作,促进采用并将业务逻辑嵌入智能AI产品。
在Artefact 工作最令人激动的事情之一是我们处理的主题的多样性。数据科学一词可以涵盖自然语言处理、预测或优化等领域的广泛技能,仅举几例,因此每项新任务都会带来新算法的测试和新技术的实验。但是,为工作挑选合适的模型并不是数据科学家的唯一责任:我们需要非常好地理解商业利害关系,以知道我们在哪里可以带来最大的价值,这意味着我们与顾问和客户密切合作。为了使我们的工作长期有用,我们还与软件工程师协调,并应用软件的最佳实践,把我们的见解变成产品。
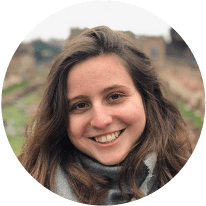
数据科学家Ombeline
一个专门的培训轨道,帮助我们的团队成长
数据科学是一个不断变化的领域,我们一心想要不断培训我们的工程师。
在Artefact ,数据科学家可以从我们培训部门精心挑选的大量内部和外部培训中受益,帮助他们达到技术前沿。
我们提供
- 机器学习培训(NLP、预测、计算机视觉、ML操作...)
- 获得云计算认证(GCP、Azure、AWS)
- 软技能培训(口头和书面陈述,谈判,项目管理)。
培训并不止于此:一个典型的数据科学家的一周都充满了学习的机会。正如我们喜欢说的 "反馈是一种礼物",我们的文化是围绕着技术活动建立的,比如我们的TechTex,在那里我们分享我们最新的项目成功和失败,或者我们的代码基地委员会(CBC),在那里我们的项目代码被我们的大师级编码员挑战
咨询公司中的科技公司
Artefact 是一家咨询公司,但DS团队首先是一个技术部门。
- 我们利用最新的模型和ML库,如Sklearn、FastAI、CatBoost、Prophet、Spacy、BERT及其变体(CamemBERT、DistilBERT...)等等。
- 我们是多云的,并且是最大的云(如GCP、Azure或AWS)的认证高级客户。
- 我们利用Docker、Kubernetes、Kedro、ML Flow、Great Expectations等ML Ops框架和工具来构建人工智能产品。
我们在团队中提倡研发,以保持与科技界的最新版本同步。
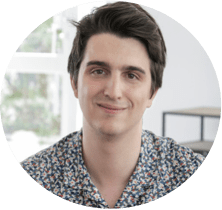
Robin Doumerc, Global CTO Artefact
近年来,我们的领域一直在不断发展,新的算法、方法和实现方式不断出现。在这个不断变化的生态系统中保持最新,如果你是一个人,可能是一项艰巨的任务。因此,作为一个团队的持续培训是我们在Artefact 的生活中必不可少的一部分,可以通过内部项目,让他们在日常遇到的问题中尝试最新的技术,或者在我们每月的培训日中专门抽出时间。让我们的数据科学家继续学习最前沿的课题,确保我们保持他们的好奇心,同时也是他们在团队中的幸福感的一部分。
加入我们
我们的团队在涉及倾向性建模和推荐系统的任务中有着良好的记录。如果你想了解更多关于我们的专业知识,请联系我们,并迅速与我们的专家取得联系。
如果你想加入我们,请关注我们的职业网页。
Medium blog articles by our tech experts
Assortment Optimization with discrete choice models in Python
Assortment optimization is a critical process in retail that involves curating the ideal mix of products to meet consumer demand while taking into account the many logistics...
Is Preference Alignment Always the Best Option to Enhance LLM-Based Translation? An Empirical Analysis
Neural metrics for machine translation (MT) evaluation have become increasingly prominent due to their superior correlation with human judgments compared to traditional lexical metrics
Choice-Learn: Large-scale choice modeling for operational contexts through the lens of machine learning
Discrete choice models aim at predicting choice decisions made by individuals from a menu of alternatives, called an assortment. Well-known use cases include predicting a...
生成式人工智能时代:正在发生的变化
The abundance and diversity of responses to ChatGPT and other generative AIs, whether skeptical or enthusiastic, demonstrate the changes they're bringing about and the impact...
How Artefact managed to develop a fair yet simple career system for software engineers
In today’s dynamic and ever-evolving tech industry, a career track can often feel like a winding path through a dense forest of opportunities. With rapid...
为什么需要大型语言模型操作 (LLMOps)
This article introduces LLMOps, a specialised branch merging DevOps and MLOps for managing the challenges posed by Large Language Models (LLMs)...
Unleashing the Power of LangChain Expression Language (LCEL): from proof of concept to production
LangChain has become one of the most used Python library to interact with LLMs in less than a year, but LangChain was mostly a library...
我们如何使用Treasure Data Unification和SQL处理配置文件命名的统一问题
In this article we explain the challenges of ID reconciliation and demonstrate our approach to create a unified profile ID in Customer Data Platform, specifically...
Snowflake的 Snowday'23:滚雪球般地取得数据科学的成功
As we reflect on the insights shared during the ‘Snowday’ event on November 1st and 2nd, a cascade of exciting revelations about the future of...
我们如何面试和聘用软件工程师Artefact
我们将详细介绍我们所寻找的技能、流程的各个步骤以及我们对所有候选人的承诺。
预测中的分类特征编码:我们都做错了吗?
我们提出了一种专门为预测应用定制的分类特征编码的新方法。
我们如何在谷歌云上部署一个简单的野生动物监测系统
我们与Smart Park合作,这是一家荷兰公司,提供先进的传感器解决方案,以保护濒危野生动物...