Author
Author
Author
In today’s digital age, organizations are challenged to keep up with the unprecedented pace of data generation and the plethora of enterprise systems and digital technologies that collect all types of data. This is coupled with the need to rapidly and efficiently analyze these large volumes of data to generate insights and intelligence in order to maximize their business value. As a result, big data platforms have become an essential foundation for organizations to efficiently deploy data solutions that provide timely data-driven business decisions and competitive advantage.
“Data analytics and intelligence solutions are proliferating across organizations to enable business growth. Organizations should build big data platforms as solid foundations to deploy data solutions at scale. These data platforms should be purpose-built for business since they are only as good as the business insights and intelligence they enable; and they should be built to be future-proof, benefiting from the constant advances in data infrastructure services and technologies.”Oussama Ahmad, Data Consulting Partner at Artefact
Key Objectives of the Big Data Platform
Big data platforms aim to break down data silos and integrate the different types of data sources required to implement advanced data analytics and intelligence solutions. They provide a scalable and flexible infrastructure for collecting, storing, and analyzing large volumes of data from multiple sources. These platforms should leverage best-in-class data management services and technologies and fulfill three key objectives:
Infrastructure of the Big Data Platform
There are several infrastructure options for a big data platform: fully on-premise, fully cloud or hybrid cloud/on-premise, each with its own advantages and challenges. Organizations should consider a number of factors when choosing the most appropriate infrastructure option for their big data platform, including data security and residency requirements, data source integrations, functionality and scalability requirements, and cost and time. A fully cloud-based architecture offers lower and more predictable costs, out-of-the-box services and integrations, and rapid scalability, but lacks control over hardware and may not comply with data privacy and residency regulations. A fully on-premise architecture provides full control over hardware and data security, typically complies with privacy and residency regulations, but incurs higher costs and requires long-term planning for scaling. A hybrid cloud/on-premise architecture offers the best of both worlds, facilitating full migration to the cloud at a later date, but may require a more complex setup.
Many organizations choose a hybrid infrastructure for their big data platforms due to organizational requirements to keep highly sensitive data (such as customer and financial data) on their own servers, or due to the lack of government-certified cloud service providers (CSPs) that meet local data privacy and residency requirements. These organizations also prefer to keep cloud-native or non-sensitive data sources in the cloud to optimize storage and compute resource costs and take advantage of out-of-the-box data analytics and machine learning services available from CSPs. Other organizations that have no organizational or regulatory requirements for data residency within the company or country opt for fully cloud-based infrastructure for faster time to implement, optimized costs, and easily scalable resources.
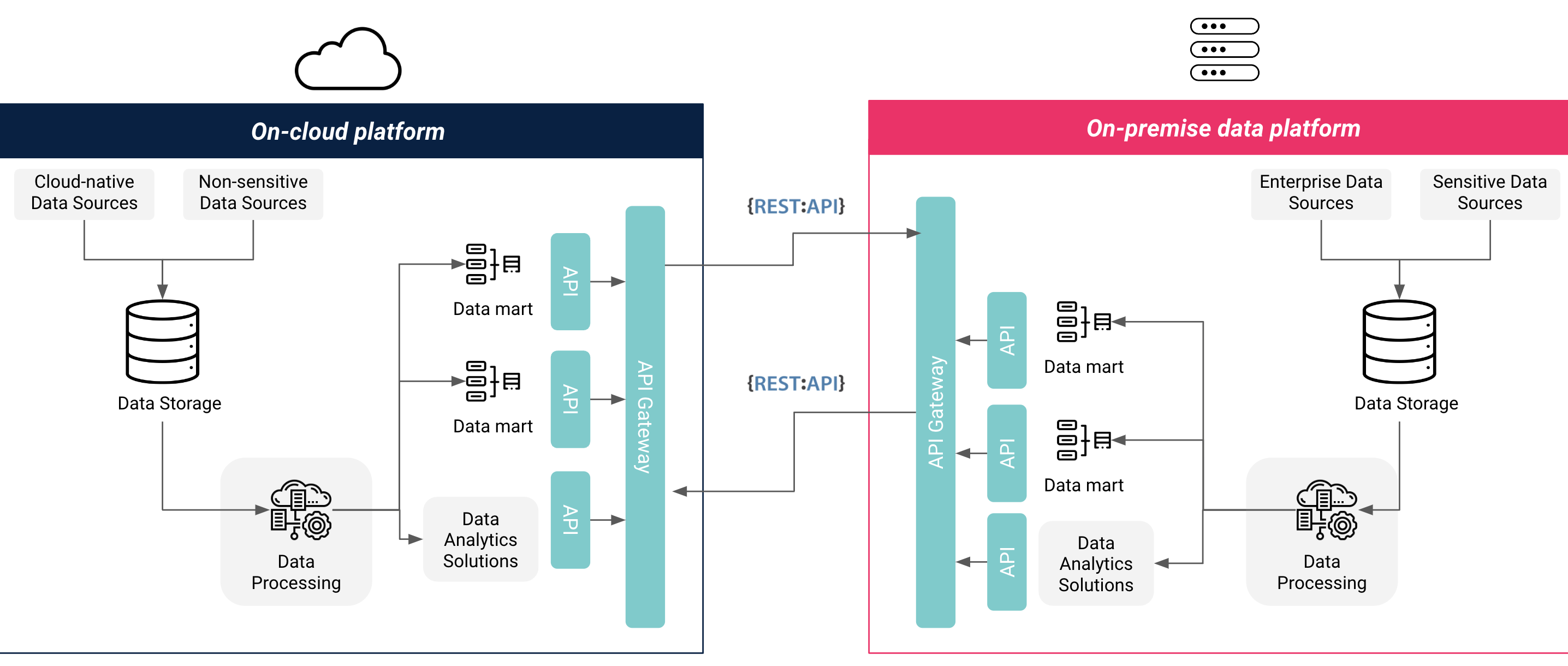
Figure 1: Hybrid Cloud & On-Premise Data Platform Infrastructure
A big data platform typically involves setting up seven main layers that reflect the data lifecycle from “raw data” to “information” to “insights”. Organizations should carefully consider the appropriate services and tools required for each of the layers to ensure seamless dataflow and efficient generation of data insights. These services and tools should perform key functions in each layer of the big data platform as shown in Figure 2: Big Data Platform Data Layers.
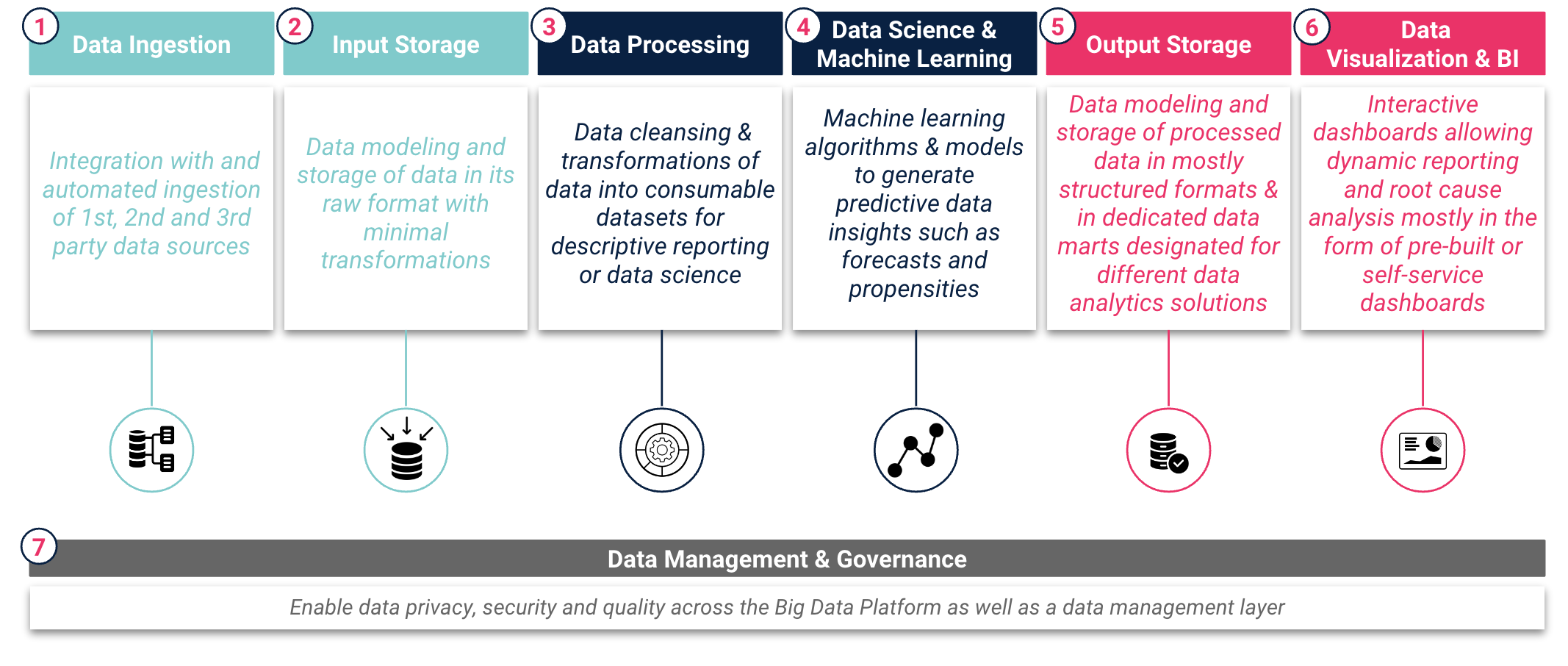
Figure 2: Big Data Platform Data Layers
Evolution of the Big Data Platform
The development of a big data platform should evolve through several stages, starting with a minimum viable platform (MVP) and continuing with incremental upgrades. An organization should synchronize the evolution of its big data platform with increased requirements for broader and faster data insights and intelligence for business decisions. These increased requirements affect the complexity of the big data platform in terms of data analytics solutions, data source volumes and types, and internal and external users. The evolution of the big data platform includes the addition of more storage and compute resources, advanced features and functionality, and improvements in platform security and management.
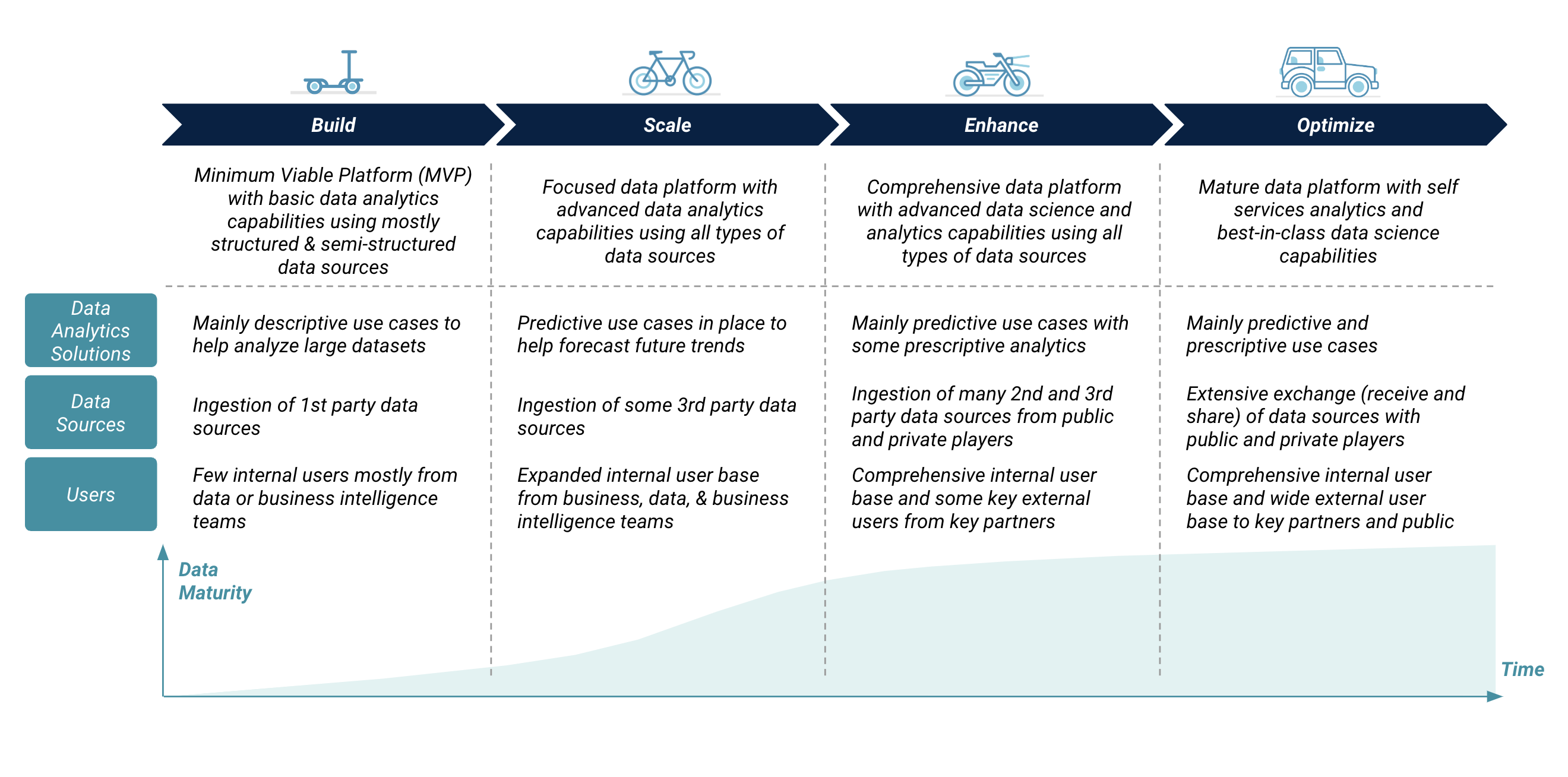
Exhibit 3: Big Data Platform Evolution
“We have seen that many organizations tend to build big data platforms with advanced and unnecessary features from day one, which increases the technology cost of ownership. Big data platform deployment should start with a minimum viable platform and evolve based on business and technology requirements. In the early stages of building the platform, organizations should implement a robust data governance and management layer that ensures data quality, privacy, security, and compliance with local and regional data laws.”Anthony Cassab, Data Consulting Director at Artefact
Guidelines for a Future-Proof Big Data Platform
A big data platform should be built according to key architectural guidelines to ensure that it is future-proof, allowing for easy scalability of resources, portability across different on-premise and cloud infrastructures, upgrade and replacement of services, and expansion of data collection and sharing mechanisms.
“An adaptable and modular platform that can scale as business needs evolve is preferable to a “black box” platform that is well integrated but allows limited customization. These platform architectures can be built fully or partially in the cloud to leverage the benefits of cloud computing, such as scalability and cost efficiency, while also meeting the privacy and security requirements of data protection regulations.”Faisal Najmuddin, Data Engineering Manager at Artefact
In summary, a big data platform brings multiple benefits to organizations, such as centralizing data sources, enabling advanced data analytics solutions, and providing enterprise-wide access to data analytics solutions and sources. However, implementing a big data platform entails a number of strategic decisions, such as choosing the right infrastructure(s), adopting a future-proof architecture, selecting standard and “migratable” services, carefully considering data protection regulations, and finally, defining an optimal evolution plan that’s closely linked to business requirements and maximizes return on data investment.
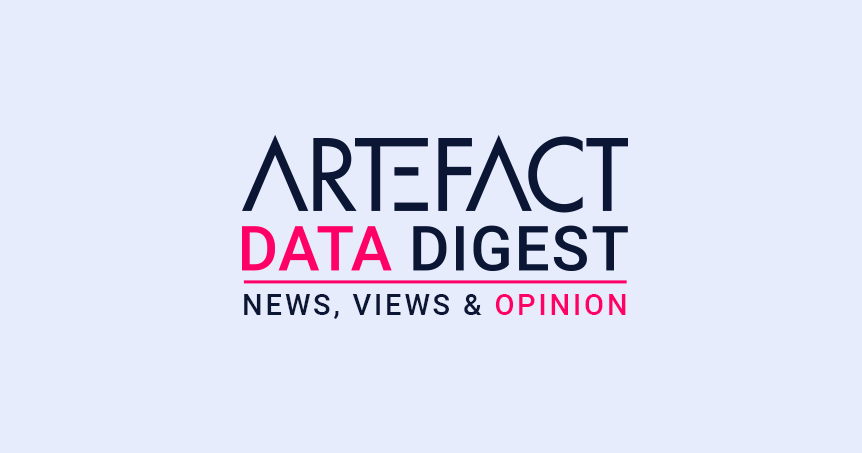
Interested in Data Consulting | Data & Digital Marketing | Digital Commerce ?
Read our monthly newsletter to get actionable advice, insights, business cases, from all our data experts around the world!