ARTEFACT Research Center
Artefact Research Center: bridging the gap between academia and industry applications.
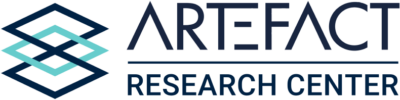
Research on more controllable, transparent and ethical models to nurture AI business adoption for the future.
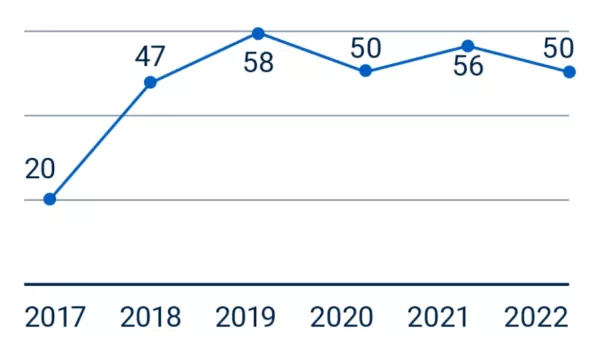
Status in business.
In recent years, AI adoption in businesses has stagnated. To illustrate, here is the share, in percentages, of respondents who say their organizations have adopted AI in at least one role.
Source: McKinsey State of AI 2022
Untrustworthy AI examples.
- AppleCard grants mortgages based on racist criteria
- Lensa AI sexualizes selfies of women
- Racist Facebook image classification with African Americans as monkeys
- Microsoft Twitter chatbot becoming Nazi, sexist and aggressive
- ChatGPT that writes a code stating good scientists are white males
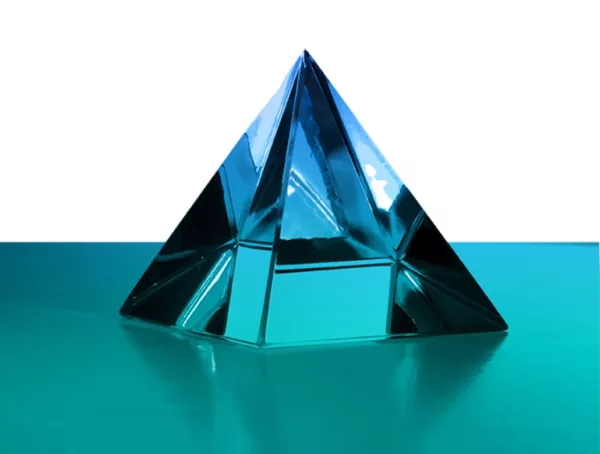
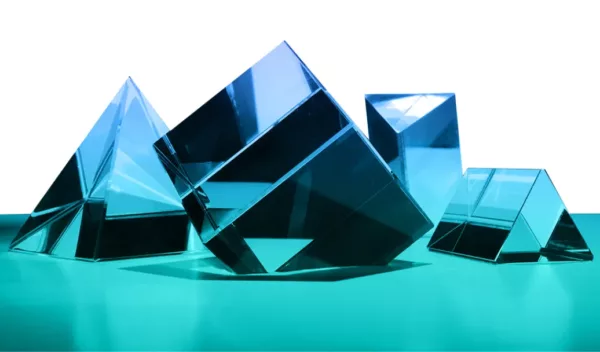
Current challenge.
AI models are accurate and easy to deploy in many use cases, but remain uncontrollable due to black boxes & ethical issues.
The Artefact Research Center’s mission.
A complete ecosystem that bridges the gap between fundamental research and tangible industrial applications.
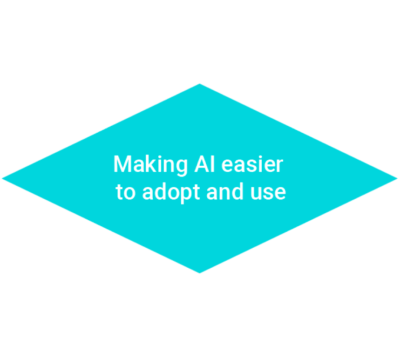
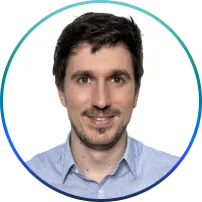
Emmanuel MALHERBE
Head of Research
Research Field: Deep Learning, Machine Learning
Starting with a PhD on NLP models adapted to e-recruitment, Emmanuel has always sought an efficient balance between pure research and impactful applications. His research experience includes 5G time series forecasting for Huawei Technologies and computer vision models for hairdressing and makeup customers at l’Oréal. Prior to joining Artefact, he worked in Shanghai as the head of AI research for L’Oréal Asia. Today, his position at Artefact is a perfect opportunity and an ideal environment to bridge the gap between academia and industry, and to foster his real-world research while impacting industrial applications.
A full ecosystem bridging the gap between fundamental research and industry tangible applications.
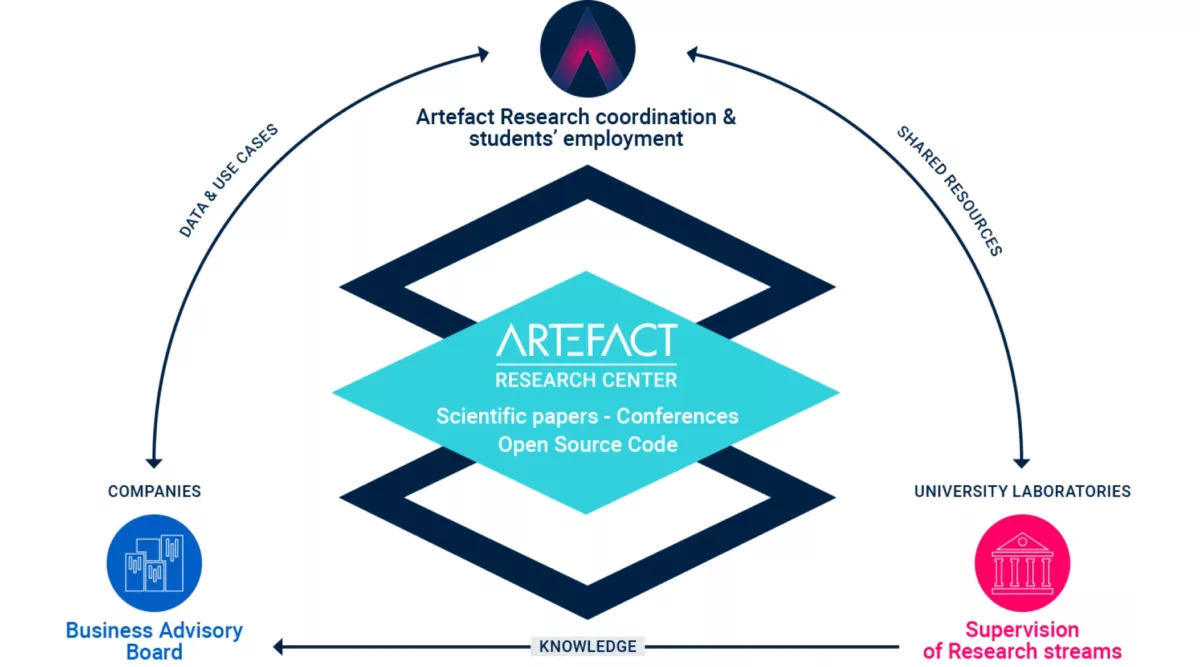
Transversal research fields.
With our unique positioning, we aim at addressing general challenges of AI, whether on statistical modelling or management research. Those questions are transversal to all our subjects and nurture our research.
Subjects.
We work on several PhD topics at the intersection of industrial use cases and state-of-the-art limitations. For each subject, we work in collaboration with university professors and have access to industrial data that allows us to address the major research areas in a given real-world scenario.
1 — Forecasting & pricing.
Model time series as a whole with a controllable, multivariate forecasting model. Such modelling will allow us to address the pricing and promotion planning by finding the optimal parameters that increase sales forecast. With such a holistic approach, we aim at capturing cannibalization and complementarity between products. It will enable us to control the forecast with guarantees that predictions are kept consistent.
2 — Explainable and controllable scoring.
A widely used family of machine learning models is based on decision trees: random forests, boosting. While their accuracy is often state of the art, such models suffer from a black-box feeling, giving limited control to the user. We aim to increase their explainability and transparency, typically by improving the estimation of SHAP values in the case of unbalanced datasets. We also aim to provide some guarantees for such models, e.g., for out-of-training samples or by enabling better monotonic constraints.
3 — Assortment optimization.
Assortment is a major business problem for retailers that arises when selecting the set of products to be sold in stores. Using large industrial datasets and neural networks, we aim to build more robust and interpretable models that better capture customer choice when faced with an assortment of products. Dealing with cannibalization and complementarities between products, as well as a better understanding of customer clusters, are key to finding a more optimal set of products in a store.
4 — AI Adoption in businesses.
The challenge of better adoption of AI in companies is to improve the AI models on the one hand, and to understand the human and organizational aspects on the other. At the crossroads of qualitative management research and social research, this axis seeks to explore where businesses face difficulties when adopting AI tools. The existing frameworks on innovation adoption are not entirely suitable for machine learning innovations, as there are typical differences with regulation, people training or biases when it comes to AI, and more so with generative AI.
5 — Data-driven sustainability.
The project will mobilize qualitative and quantitative research methods and address two key questions: How can companies effectively measure social and environmental sustainability performance? Why do sustainability measures often fail to bring about significant changes in organizational practices?
On the one hand, the project aims to explore data-driven metrics and identify indicators to align organizational procedures with social and environmental sustainability objectives. On the other hand, the project will focus on transforming these sustainability measures into concrete actions within companies
6 — Bias in computer vision.
When a model makes a prediction based on an image, for instance showing a face, it has access to sensitive information, such as the ethnicity, gender or age, that can bias its reasoning. We aim at developing a framework to mathematically measure such bias, and propose methodologies to reduce this bias during the model training. Furthermore, our approach would statistically detect zones of strong bias to explain and understand and control where such models reinforce the bias present in the data.
7 — LLM for information retrieval.
One major application of LLMs is when they are coupled with a corpus of documents, which represent some industrial knowledge or information. In such a case, there is a step of information retrieval, for which LLMs show some limitations, such as the size of the input text, which is too small for indexing documents. Similarly, the hallucination effect can also happen in the final answer, which we aim at detecting using the retrieved document and model uncertainty at inference time.
Artefact’s part-time researchers.
Besides our team dedicated to research, we have several collaborators who spend some of their time doing scientific research and publishing papers. By also working as consultants, they are inspired by real-world problems encountered with our clients.